We develop electronic structure descriptors and machine-learning algorithms to chart the materials space and speed up first-principles simulations of complex properties (see npj Computational Materials volume 10:278 (2024)). By coupling machine-learning methods with physics-based models, we target accurate predictions of electronic structure properties at low cost. The combination of disclosing unknown patterns in the materials space with data mining and the speed-up in the prediction of materials properties through machine learning accelerates the design and discovery of novel materials.
Machine Learning
Predicting electronic structure properties
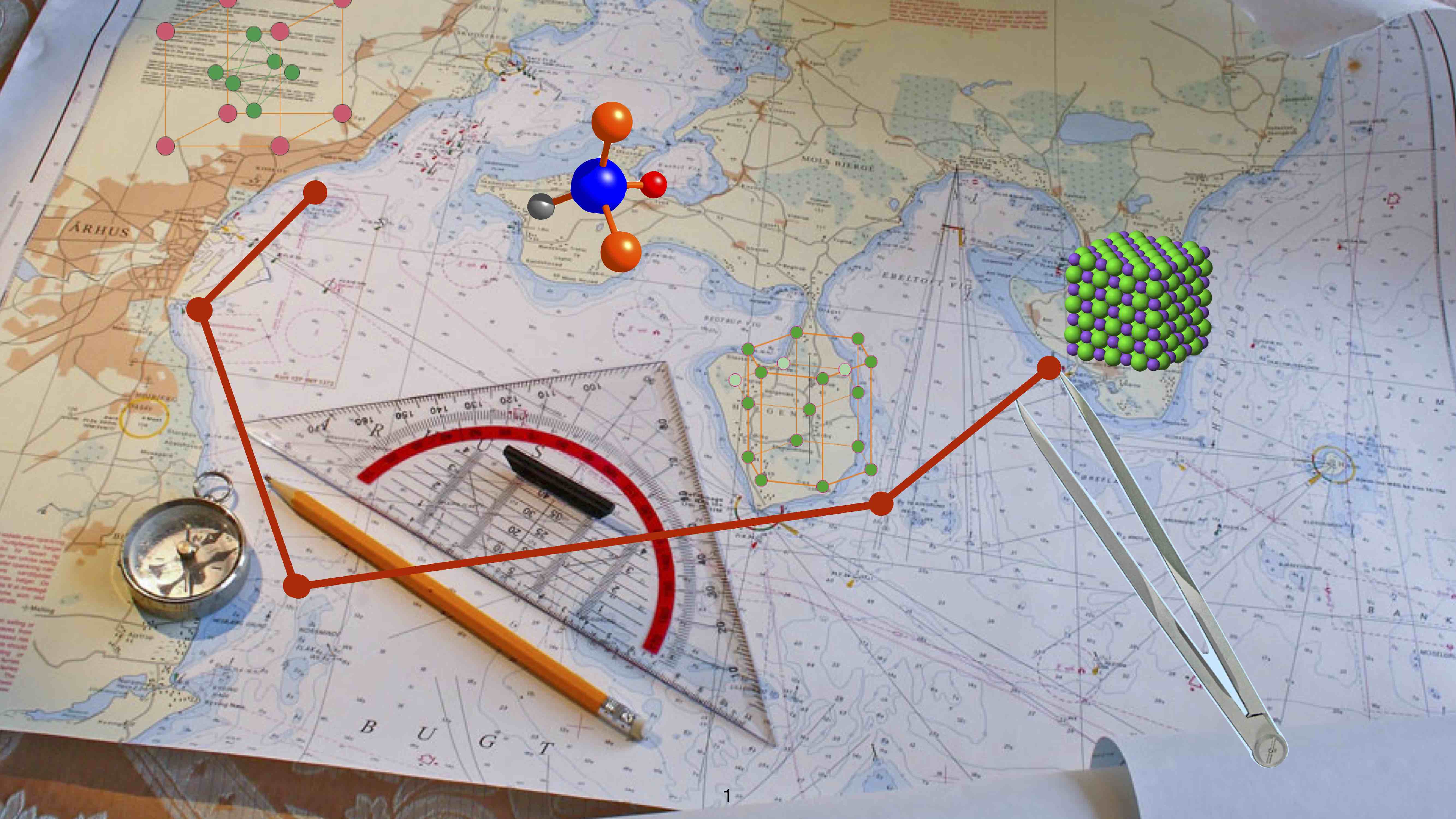
Neural-network interatomic potential for materials
We are building classical interatomic potential based on neural-network architectures to study anharmonic effects and finite-temperature properties of materials. The training is powered by ab-initio molecular dynamics, exploiting modern GPU-accelerated supercomputers to tackle large systems.